Modular Sample Replacement: Overview
This first application uses a supervised ML classification algorithm to analyze and resynthesize a drumbeat.
Audio I: An eight-measure-long drum beat. The input audio for our application.
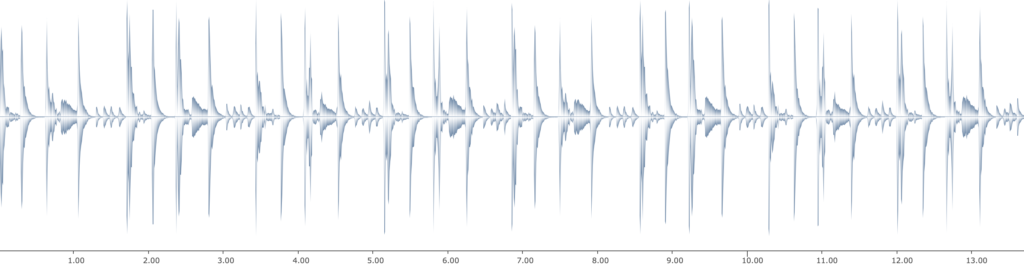
Figure 4: An eight-measure-long drum beat. The input audio for our application.
For a demonstration of the application, we have an eight-measure-long drum beat of Mark Giuliana (see figure 4). The drumbeat is automatically segmented into individual transients using OM-superVP and OM-SoX.
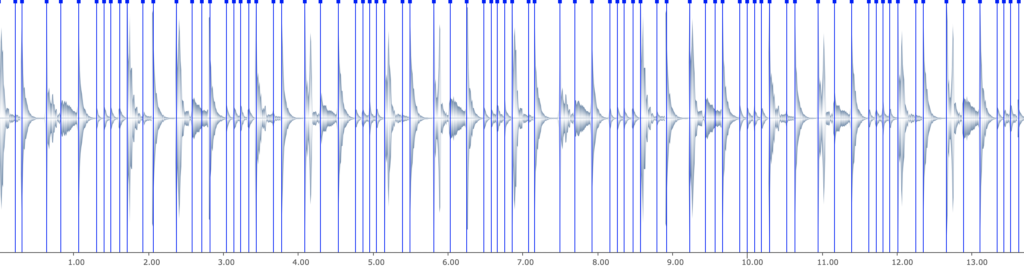
Figure 5: The audio is segmented into individual transients
Then, each individual segment is compared against a training set of audio, and then assigned a class-id that designates what instrument type it is (i.e. a kick, a cymbal, a snare-drum). This training set is 105 samples of drum hits (15 samples each of open cymbals, hi-hats, snares (snare on), snares (snare off), cross-sticks, toms, and kicks).
The segments are then notated onto a multi-seq, an OpenMusic score object for multi-staff western music notation), with each class-id sorted into its own stave.
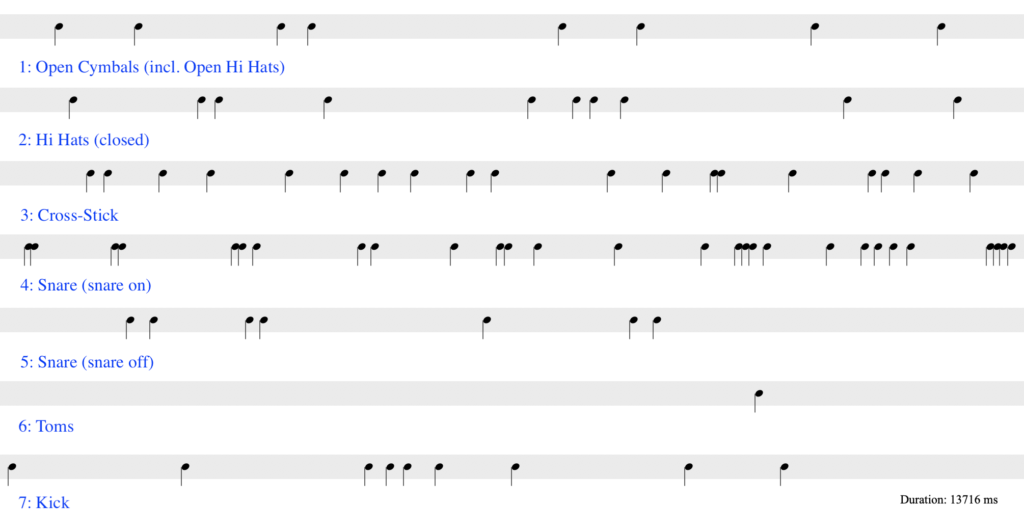
Figure 6: Each segment of the audio is classified and notated on a polyphonic score object
The multi-seq acts as a score from which OM-SoX synthesizes a new sound with replacement samples. The multi-seq could theoretically be left unchanged, facilitating a simple sample replacement. Or, the multi-seq could be further edited by user-data to create a wholly new sound, merely informed by the original drum beat. The replacement sample engine can incorporate any number of replacement sample libraries, calling from one or all of them throughout the sound synthesis process.
In our demonstration, there are three sample replacement libraries: an acoustic drumset, an electronic drumset, and a set of field recordings. The audio below is our original drum beat is reconstructed with samples from an electronic drumset
This next sound (Audio III) is the exact same multi-seq, only with the samples interpolating between three different sample sets (acoustic, electronic, synth).
Finally, here in Audio IV is the drum-beat reconstructed, but first with the chord-seq being edited, yielding an entirely new drum beat.
The sample replacement engine is controlled by a BPF-library, a set of break point functions (BPFs) equal to the number of samples called by the multi-seq. Each BPF is used to create a weight-vector that influences what sample is called. In figure 8, this BPF below determines the likelihood of a given sample set being called. Additionally, the BPF allows for a weight-vector to be called regardless of how many sample sets are at play.
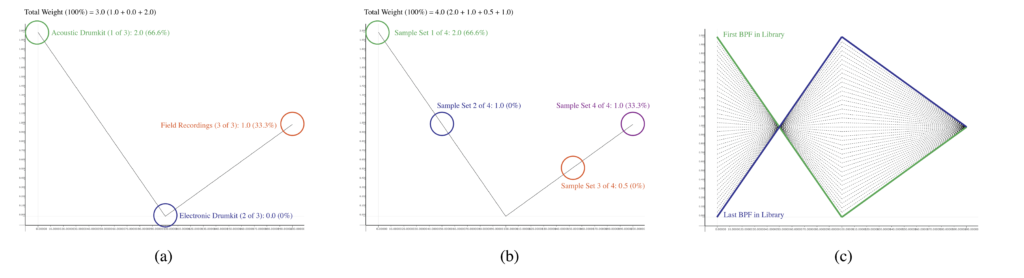
Figure 7: (a) and (b) illustrate how the replacement of a single given sound would be weighted according to whether there were three or four sample sets involved in the synthesis process. (c) is a BPF-library holding all of the BPFs handling each sample. In (c), there is a gradual interpolation from one sample replacement weighted vector to another.
In Audio IV, the synthesis begins with mainly acoustic drum samples, as well as some field recordings. Then gradually, the acoustic drum samples transition to electronic drum samples, while the field recordings remain. This is because the BPF-library used gradually interpolates between the first and last BPF (see figure 7c).
About the author